Abstract
Free full text

Tracking SARS-CoV-2 mutations and variants through the COG-UK-Mutation Explorer
Abstract
COG-UK Mutation Explorer (COG-UK-ME, https://sars2.cvr.gla.ac.uk/cog-uk/—last accessed date 16 March 2022) is a web resource that displays knowledge and analyses on SARS-CoV-2 virus genome mutations and variants circulating in the UK, with a focus on the observed amino acid replacements that have an antigenic role in the context of the human humoral and cellular immune response. This analysis is based on more than 2 million genome sequences (as of March 2022) for UK SARS-CoV-2 data held in the CLIMB-COVID centralised data environment. COG-UK-ME curates these data and displays analyses that are cross-referenced to experimental data collated from the primary literature. The aim is to track mutations of immunological importance that are accumulating in current variants of concern and variants of interest that could alter the neutralising activity of monoclonal antibodies (mAbs), convalescent sera, and vaccines. Changes in epitopes recognised by T cells, including those where reduced T cell binding has been demonstrated, are reported. Mutations that have been shown to confer SARS-CoV-2 resistance to antiviral drugs are also included. Using visualisation tools, COG-UK-ME also allows users to identify the emergence of variants carrying mutations that could decrease the neutralising activity of both mAbs present in therapeutic cocktails, e.g. Ronapreve. COG-UK-ME tracks changes in the frequency of combinations of mutations and brings together the curated literature on the impact of those mutations on various functional aspects of the virus and therapeutics. Given the unpredictable nature of SARS-CoV-2 as exemplified by yet another variant of concern, Omicron, continued surveillance of SARS-CoV-2 remains imperative to monitor virus evolution linked to the efficacy of therapeutics.
1. Introduction
As of March 2022, SARS-CoV-2, the causative agent of COVID-19, has accounted for over 450 million infections and 6 million deaths worldwide (https://covid19.who.int/). SARS-CoV-2 was first identified at the end of 2019 in the city of Wuhan, China, and has since spread with unprecedented efficiency among humans (Hu et al. 2021). In contrast to other RNA viruses, the Coronaviridae family is characterised by relatively high-replication fidelity due to the proofreading activity of their polymerases (Robson et al. 2020). Early analyses of SARS-CoV-2 genomes estimated an evolutionary rate of around 0.001 subs/site/year (two to three mutations per month) (Duchene et al. 2020); however, there is much deviation from this rate across the phylogeny with several outlier lineages,Appendix 1 including variants of concern (VOCs), that have rapidly acquired several mutations at a much higher rate than this. The analysis of mutations from virus genome data is important for basic virology (Houldcroft et al. 2017), to identify evolutionary signals associated with mutations prior to experimental and real-world data on clinical outcomes or vaccine effectiveness, and to document and track changes that could alter the effectiveness of therapeutics. At present, almost 9 million genome sequences are now available via the GISAID Initiative, permitting near real-time surveillance of the unfolding pandemic (Shu and McCauley 2017; Meredith et al. 2020).
SARS-CoV-2 showed relatively inconsequential genetic change until late 2020 (MacLean et al. 2021). Subsequently, later months of 2020 were characterised by the emergence, across the globe, of VOCs possessing mutations that altered virus phenotype in terms of transmissibility and antigenicity (Harvey et al. 2021). Concurrently, shifts in the immune profile of the human population likely represented a change in the selective environment evidenced by an increase in dN/dS ratios indicative at positive selection at codons across the genome and notable levels of convergence across the global phylogeny (Martin et al. 2021). The continuing emergence of SARS-CoV-2 variants exhibiting heightened transmissibility or antigenic novelty necessitates tools to detect, describe, and track those antigenic changes and make this information accessible to researchers, public health agencies, and drug and vaccine developers so that the information becomes actionable.
Since the beginning of the pandemic, several bioinformatics tools have been developed to analyse and generate outputs that support actionable information (e.g. Pangolin lineages https://cov-lineages.org/index.html; https://filogeneti.ca/covizu/; https://outbreak.info; COVID-19 CG https://covidcg.org; https://coval.ccpem.ac.uk/; CoV-GLUE http://cov-glue.cvr.gla.ac.uk, https://nextstrain.org; and https://covariants.org—last accessed date: 16 March 2022). Although these tools have been essential for data curation, analysis research, and public health impact (Hufsky et al. 2021), they have been mainly focusing on the epidemiological aspects of the pandemic, lacking the relevant information from the literature on the immunological effect of mutations.
This scientific need led us to create the COG-UK-Mutation Explorer (COG-UK-ME), a web
resource that provides tracking of non-synonymous mutations in SARS-CoV-2 genome. COG-UK-ME
is based on UK data, and it has been developed by the COVID-19 Genomics UK (COG-UK)
consortium—created to deliver large-scale and rapid whole-genome virus sequencing to local
National Health Service centres and the UK government. COG-UK-ME relies on CLIMB-COVID, a
data-centric bioinformatics environment for centralising UK SARS-CoV-2 sequences (Nicholls et al. 2021a). Here, we describe COG-UK-ME and
its main functionality. COG-UK-ME currently has around 5,000 users per month, with
approximately 30per cent from the UK, 20
per cent from the USA, and the remainder from
other international locations.
COG-UK-ME has three aims: first, to make available amino acid mutations in a user-friendly way, enabling data transparency; second, to report on amino acid variation present in SARS-CoV-2 sequences that have been shown to confer resistance against antibodies or disrupt T cell epitope binding. The third is to report on the emergence of new mutations that have the potential to reduce the effectiveness of some therapeutics that have been granted approval for use. Data accumulating over a time course can be analysed so that trends can be detected and tracked.
2. Data analysis
COG-UK-ME is a publicly accessible web resource that displays in-depth information and analyses of SARS-COV-2 virus genome mutations and variants. Sequence information is deposited daily on the MRC CLIMB-COVID platform (Nicholls et al. 2021a), which has been generated by the COG-UK Consortium, Wellcome Sanger Institute, public health agencies, and other approved providers. Virus lineages are assigned by using a phylogenetic framework to identify those lineages that contribute most to active spread (Rambaut et al. 2020; O’Toole et al. 2021). Mutations for UK sequences are then analysed on the CLIMB platform and linked with curated data on antigenicity, therapeutics, and drug resistance. The prepared data files are then transferred from CLIMB to a web server and visualised.
2.1. Tracking changes in the mutation count
COG-UK-ME shows a browsable dataset of all the amino acid sequence variations in
SARS-CoV-2 protein sequences. These are shown for all data, and in the recent past—over
the last 28days—in the UK and in the four UK nations (England, Scotland, Wales, and
Northern Ireland) (‘Mutation Counts’ and ‘Mutations by week’ tabs). The ‘VOCs and VUIs in
the UK’ tab shows through tables and visualisations the number of sequences of variants
under investigation (VUI) and VOCs as designated by the UK Health Security Agency
(formerly Public Health England) (https://www.gov.uk/government/publications/covid-19-variants-genomically-confirmed-case-numbers/variants-distribution-of-cases-data—last
accessed date: 16 March 2022) (Fig. 1A). COG-UK-ME
also provides visualisations of the spike protein structure showing the position of the
VOC-defining mutations (Fig. 1B). Data are also
placed in their geographical context by showing the number of sequences and percentage of
variants per region (Nomenclature of Territorial Units for Statistics—NUTS1)
(‘Geographical distribution’ tab).
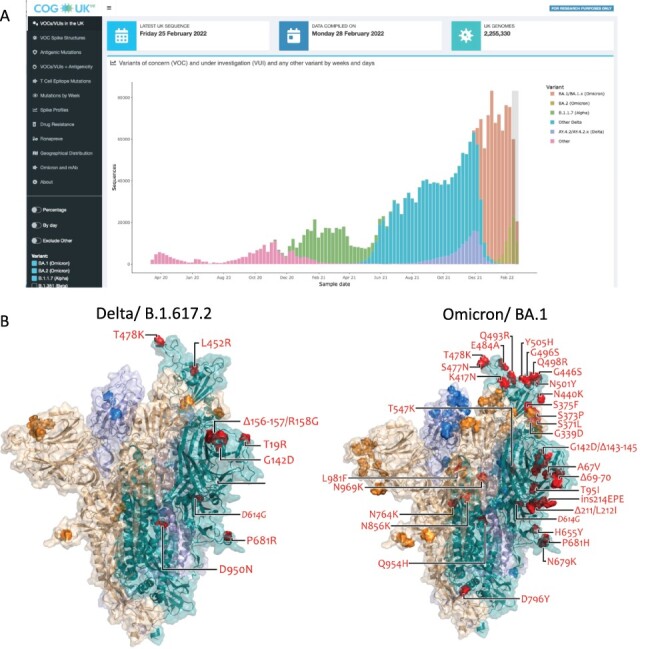
(A) Frequency plot showing the number of SARS-CoV-2 sequences per week for VOCs Alpha, Delta, Delta-AY4.2, Omicron BA.1 and BA.2, and ‘other’ pre-VOC variants (see key) in the UK. The light grey box covering the two most recent weeks indicates a period in which sequence counts are low due to a lag (Figure S1). (B) Spike protein structure showing locations of Delta- and Omicron-specific spike mutations. Ectodomain of the spike homotrimer in open conformation with individual spike protein chains shown in different colours. On each monomer, highlighted spheres show the locations of amino acid substitutions, deletions (Δ), or insertions (ins) that distinguish the Omicron (BA.1) variant, relative to the original genotype (Wuhan-Hu-1). These are annotated on the monomer with an ‘up’ receptor-binding domain where they are highlighted in red on teal. The substitution D614G, which is shared by common descent by all lineage B.1 descendants is italicised. The visualisation is made using a complete spike model (Woo et al. 2020), which is in turn based upon a partial cryo-EM structure (RCSB Protein Data Bank (PDB) ID: 6VSB (Wrapp et al. 2020)).
2.2. Spike profile tracking
In addition to tracking the frequency of individual substitutions across the genome and
of lineages identified as VOCs or VUIs, changes in the frequency of combinations of spike
amino acid substitutions are tracked. Each spike profile is defined as the combination of
substitutions compared with the original genotype (Wuhan-Hu-1). Profiles may represent
monophyletic lineages or they may have arisen convergently across the phylogeny. Changes
in profile frequency over the latest 56-day period are considered. For currently
circulating profiles (those sampled within the latest 7 days), a sortable and searchable
table includes information on the pango lineage(s) for which the profile has been
associated, the number of substitutions comprising the profile, and the count of sequences
across the latest 56-day and 28-day periods. The average growth rate (plotted on the
y-axis in Fig. 2A) is calculated as the mean
percentage change in frequency between each 2-week period within the 56-day period. As
growth rates are sensitive to potentially stochastic changes at very low frequencies, we
also calculate a statistic that estimates recent expansion or contraction of each profile,
calculated over the 56-day period (plotted on the y-axis in Fig. 2B). For each profile, , the
absolute value for this statistic,
, is calculated
using the observed frequency,
, of each
profile,
, in each of the most recent 2-week
periods,
, according to
where is the frequency of profile
over the full 8-week period under
consideration. Thus, the value calculated is influenced by both the rate of change in
profile frequency and the overall frequencies of a given profile and is more robust to
stochastic differences in profile frequency that tend to occur at low frequencies.

Spike profiles sampled within 7days of the latest UK sequence are summarised. Each
spike profile is a set of amino acid substitutions listed relative to the original
genotype (Wuhan-Hu-1). Figure prepared with data compiled on 27 February 2022 with a
most recent sequence date of 24 February 2022. A) Points represent spike profiles
positioned by the number of sequences in the latest 28-day period and the average
growth rate calculated over the latest 56-day period. Points are coloured by an
expansion/contraction statistic that takes both the rate of change in frequency and
the overall frequencies of a profile into account. The cursor is hovering to show
information associated with BA.2
+
P1162L. B) Points represent spike profiles
positioned by the number of sequences in the latest 28-day period and the
expansion/contraction statistic used to colour points in A. Here, points
are coloured to show profiles associated with Delta, Omicron (BA.1/BA.1.x), and
Omicron (BA.2) variants. The cursor is again positioned to highlight the position of
BA.2
+
P1162L. The plot has been zoomed to focus on profiles with 28-day counts
between 100 and 1,000. C) Searchable table sorted to show the four profiles in the UK
with the highest values in the expansion/contraction column. Further columns show
profile numbers in the latest 28- and 56-day periods and average growth rate.
This monitoring of spike profiles allows the detection of emerging, potentially
advantageous, spikes that might not be detected by surveillance methods conditioned on
mutations previously determined to be noteworthy through experimentation or other means.
This simple approach is complementary to more sophisticated phylogenetic approaches for
the estimation of lineage-specific growth rates. One advantage of this simple
non-phylogenetic approach is that the convergent accumulation of a substitution or
combination of substitutions on a particular background is identified. Such a scenario
could arise when there is strong selective pressure on a genotype (e.g. the introduction
of a therapeutic). For example, this approach would quickly alert to the growth of a
profile such as Delta+
E484K emerging convergently across the Delta phylogeny in response
to within-host, immune-mediated selection, even if the instances of this profile are
interspersed across the phylogeny.
2.3. Antigenic changes
The ‘Antigenic changes’ tab shows a table listing all mutations in the spike protein present in the UK sequence dataset that have individually been associated with some significant degree of weaker virus neutralisation by convalescent plasma, post-vaccination sera, or SARS-CoV-2 spike-specific mAbs (referred to as ‘Escape mutations’ in Fig. 3). Alongside links to the associated literature for each substitution, a confidence score representing the weight of evidence associated with each substitution is shown: ‘high’, whenever the antigenic role of mutation is supported by multiple studies, including at least one that reports an effect observed with (post-infection serum) convalescent plasma; ‘medium’, if the antigenic role of the mutation is supported by multiple studies; and ‘low’, when the mutation is supported by a single study (Fig. 3). In the ‘VOCs + Antigenicity’ tab, COG-UK-ME reports the occurrence of additional amino acid substitutions or deletions linked to antigenic change within each VOC (Fig. 4). Relative proportions (expressed as percentages) of sequences carrying specific mutations can give information about the antigenic diversity within a VOC lineage.

Amino acid substitutions in the spike protein identified in the UK dataset (referred to as ‘Escape mutations’) that have been associated with weaker neutralisation of the virus by convalescent or post-vaccination plasma/serum or spike-specific monoclonal antibodies (mAbs) or that have been observed to emerge upon exposure to either mAbs or plasma in laboratory experiments.

Heatmap showing the frequency of spike amino acid substitutions and a deletion with a potential or confirmed antigenic role on top of BA.2 through time. The labelled structural domains are indicated on the left side: SP, signal peptide; NTD, N-terminal domain; RBD, receptor-binding domain; RBM, receptor-binding motif; S2, subunit; FP, fusion peptide. Residues are also coloured according to the class of antibody that binds to an epitope. RBD antibody Classes 1–4 (Barnes et al. 2020) are depicted by colours: green (Class 1: ACE2 blocking, bind open RBD only), yellow (Class 2: ACE2 blocking, bind open, and closed RBD), blue (Class 3: non-ACE2 blocking, bind open, and closed RBD), or yellow (Class 4: non-ACE2 blocking, bind open RBD only). Residues described in an NTD epitope (Chi et al. 2020) are coloured in magenta (Class 5). Each residue is also classified as having evidence for mutations either affecting neutralisation by mAbs (Baum et al. 2020; Li et al. 2020; Weisblum et al. 2020; Liu et al. 2021) or serum from previously infected individuals (convalescent plasma) (Li et al. 2020; Weisblum et al. 2020; Andreano et al. 2021; Greaney et al. 2021; Liu et al. 2021) or vaccinated individuals (Wang et al. 2021) and emerging upon exposure to mAbs (Baum et al. 2020; Weisblum et al. 2020; Liu et al. 2021) or plasma (Weisblum et al. 2020; Andreano et al. 2021) in laboratory experiments.
2.4. T cell epitope mutations
Similar to the ‘Antigenic changes’ tab, the ‘T cell epitope mutations’ tab shows amino acid replacements in experimentally proven T cell epitopes both in spike and in other proteins, which have been described in the literature. Data are further filtered based on experimental studies just defining T cell epitopes (‘Epitope studies’) or those reporting on the impact of specific mutations on T cell recognition (‘Reduced T cell recognition’). Also shown are predicted antigen presentation likelihood percentile rank values to the experimentally proposed HLA restriction element based on the NetMHCpan (CD8) and NetMHCIIpan (CD4) 4.1 algorithms (https://services.healthtech.dtu.dk/service.php?NetMHCpan-4.1 and https://services.healthtech.dtu.dk/service.php?NetMHCIIpan-4.0—last accessed date: 16 March 2022) (Reynisson et al. 2020) for both the wild-type and mutant peptide variants. Here, peptides with predicted percentile rank scores of less than 2.0 for CD8 and less than 5.0 for CD4 are likely HLA binders. Amino acid replacements in any epitope are visualised through logo plots, in which each letter represents an amino acid replacement present in a specific epitope, and its height represents residue frequency. The number below the sequence logo shows the position relative to the start position of the epitope.
2.5. Drug resistance
The ‘Drug resistance’ and ‘Ronapreve’ tabs show tables and visualisations for those mutations associated with the resistance of SARS-CoV-2 to antiviral treatments (e.g. Remdesivir) and therapeutic mAb cocktails that are currently used in clinical settings (e.g. Ronapreve, cocktail of casirivimab and imdevimab) (Beigel et al. 2020; Sidebottom and Gill 2021). The UpSet plot in the Ronapreve tab allows users to track amino acid substitutions known to affect either casirivimab or imdevimab mAbs and in combination (Fig. 5). Other therapeutics will be added in the future.

UpSet plot showing the counts of mutations affecting Ronapreve constituent mAbs that
have occurred individually and in combinations (Lex
et al. 2014). Occurrence is shown in the full UK SARS-CoV-2 genome sequence
dataset (A) and in a dataset compiled of sequences in the latest 28-day period (B).
Spike amino acid substitutions known to affect either casirivimab or imdevimab mAbs
were considered. The upper histogram shows the number of sequences per mutation (dots)
or combination of mutations (lines), and the bottom left histogram presents the number
of sequences with each specific substitution. Rows are coloured according to the mAb
to which the greatest fold decrease in binding was recorded (blue=
casirivimab,
orange
=
imdevimab), with a lighter shade indicating a fold decrease of less than 100
and darker shade indicating 100 or greater.
3. Concluding remarks
Bioinformatics resources such as COG-UK-ME play an important role by providing clear and accessible information to those who are tackling the pandemic, including through public health actions and the development of vaccines and therapeutics. COG-UK-ME is unique in presenting data from a densely sequenced population with an emphasis on publicly available data (bioproject accession PRJEB37886 and public alignments https://www.cogconsortium.uk/tools-analysis/public-data-analysis-2/—last accessed date: 16 March 2022). COG-UK-ME also brings together curated literature on the impact of mutations on various functional aspects of the virus. The COG-UK-ME interface allows users to track mutations that are a potential threat based on a phenotypic impact on virus biology or by conferring resistance to the human immune response, including that boosted by vaccines or antiviral drugs. Rapid analyses of VOCs, e.g. the accumulation of any mutation, can also be obtained from the COG-UK-ME interface. Of particular interest to researchers and for therapeutics are mutations that either have an antigenic role or affect T cell binding. These mutations are intensely monitored by researchers and Public Health Agencies to identify any new variant that could escape the immunity generated by vaccines. Timely identification of VOC/VUI samples can facilitate access to clinical specimens to isolate live virus and serum for further immunological evaluation.
Although amino acid sequence analyses are not sufficient to determine the functional effect of a single mutation on SARS-CoV-2 fitness when taken in isolation, COG-UK-ME strives to collate all the available literature on SARS-CoV-2 mutations and provides data to support experiments that investigate the change in phenotype that these mutations might confer on variants.
4. Methods
Throughout COG-UK-ME, Wuhan-Hu-1 (NCBI RefSeq NC_045512) is used as the reference sequence for nucleotide coordinates, codon numbering within viral proteins, and wild-type amino acid assignments. Sequences are regularly uploaded onto the MRC-CLIMB platform. Sequences with quality issues are excluded. Amino acid replacements and in-frame indels in each sequence are identified (Nicholls et al. 2021a).
Source code is available at https://github.com/wrightdw/COG-UK-ME (last accessed date: 16 March 2022).
4.1. Data preparation
Sequence metadata files are processed on the CLIMB-COVID platform (Nicholls et al. 2021a) using the R statistical programming language (Team 2021) and the Tidyverse collection of R packages (Wickham et al. 2019). Non-UK sequences are filtered out. Amino acid replacements and reference amino acids are counted for all times and for a 28-day period up to and including the latest sequence date for the UK and the four UK nations. Counts are linked with data on antigenic changes, data on therapeutics, epitope data and predicted epitope binding percentile rank values. Counts of all amino acids across all positions in the spike protein are prepared for the visualisation of sequence logos.
PANGO lineage name aliases are resolved to the full lineage names using the current designations at https://github.com/cov-lineages/pango-designation (last accessed date: 16 March 2022). VOC and VUI lineages are counted by day and by week for the UK and the four UK nations, counting AY.x sub-lineages within the Delta VOC hierarchically. VOC lineages are also counted by week and by geographic region according to the 12 Nomenclature of Territorial Units for Statistics first-level regions of the UK (NUTS1). Antigenic amino acid replacements and deletions in the spike protein are counted for VOC lineages, excluding lineage defining replacements, as defined by the UK Health Security Agency at https://github.com/phe-genomics/variant_definitions (last accessed date: 16 March 2022). Following data preparation, the resultant data files are transferred from CLIMB to a web server for visualisation.
4.2. Literature search
We searched PubMed, LitCovid, BioRxiv, and MedRxiv using the search term ‘SARS-CoV-2’ combined with ‘mAbs’, ‘monoclonal’, ‘convalescent’, ‘neutralisation/neutralization’, ‘epitope’, and ‘antibody’ for studies published from January 2020 to July 2021 and manually searched the references of select articles for additional relevant articles (Figure S2). We also searched BioRxiv, and MedRxiv using combinations of the search terms: ‘COVID19’, ‘COVID-19’, ‘SARS-CoV-2’, ‘remdesivir’, ‘favipiravir’, ‘molnupiravir’, ‘nirmatrelvir’, ‘ritonavir’, ‘paxlovid’, ‘antiviral’, ‘binding’, ‘efficacy’, ‘effective’, ‘resistance’, ‘resistant’, ‘sensitivity’, ‘inhibit’, ‘evasion’, ‘mutation’, and ‘variant’. Results reporting on SARS-CoV-2 mutations that cause resistance to antiviral drugs were recorded and published on the dashboard. This included many different types of assays and studies: neutralisation assays, receptor binding assays, clinical efficacy studies, transcriptional inhibition assays, and in silico indications of resistance. Antiviral drugs are included in the review if they are clinically approved somewhere in the world or are in Stage 3 clinical trials. This search is repeated each week, allowing the timely updating of the dashboard when new research arises.
4.3. Data visualisation
The Shiny framework is used to create the COG-UK-ME web application, hosted in the Shiny Server environment (Chang et al. 2021). In order to maximise performance across multiple concurrent users, most values are pre-computed in the data preparation process on CLIMB, with the web application focussing on data visualisation.
The bar charts for VOC lineages and mutations, the geographical maps of VOC lineages, and the scatter plot of spike profiles are generated using ggplot2, with interactive features added using Plotly (2015). The heatmap of antigenic changes in the spike protein is generated using the ComplexHeatmap package (Gu et al. 2016), antigenic replacements, and structural domain classifications. Amino acid replacements in epitopes are visualised as sequence logos using the ggseqlogo package (Wagih 2017). UpSet plots for mutations affecting Ronapreve are generated using the UpsetR package (Lex et al. 2014; Conway et al. 2017). The web application user interface is created using the shinydashboard (Chang and Ribeiro 2021), shinydashboardPlus (Granjon 2021), shinyWidgets (Perrier et al. 2021), and shinyjs packages (Attali 2020).
For the visualisations of the VOC spike mutations on the structure, the file 6vsb_1_1_1.pdb containing a complete model of the full-length glycosylated spike homotrimer in open conformation with one monomer having the receptor-binding domain in the ‘up’ position was obtained from the CHARMM-GUI Archive (Woo et al. 2020; CHARMM-GUI Archive, 2021). This model is itself generated based upon a partial spike cryo-EM structure (PDB ID: 6VSB (Wrapp et al. 2020)). For visualisation, the model was trimmed to the ectodomain (Residues 14–1164) and the signal peptide (Residues 1–13) and glycans were removed. Figures were prepared using PyMol (Schrödinger-LLC 2010).
Acknowledgements
COG-UK-ME is enabled by data from COG-UK and MRC-CLIMB. All UK data are available in GISAID, and we thank all sequence generators for rapid data-sharing. We also acknowledge Omar Wagih for his support with his ggseqlogo sequence logo package used for visualising mutations in epitopes.
Appendix
The COVID-19 Genomics UK (COG-UK) consortium June 2021 V.1
Funding acquisition, Leadership and supervision, Metadata curation, Project administration, Samples and logistics, Sequencing and analysis, Software and analysis tools, and Visualisation:
Dr Samuel C Robson 13, 84
Funding acquisition, Leadership and supervision, Metadata curation, Project administration, Samples and logistics, Sequencing and analysis, and Software and analysis tools:
Dr Thomas R Connor 11, 74 and Prof Nicholas J Loman 43
Leadership and supervision, Metadata curation, Project administration, Samples and logistics, Sequencing and analysis, Software and analysis tools, and Visualisation:
Dr Tanya Golubchik 5
Funding acquisition, Leadership and supervision, Metadata curation, Samples and logistics, Sequencing and analysis, and Visualisation:
Dr Rocio T Martinez Nunez 46
Funding acquisition, Leadership and supervision, Project administration, Samples and logistics, Sequencing and analysis, and Software and analysis tools:
Dr David Bonsall 5
Funding acquisition, Leadership and supervision, Project administration, Sequencing and analysis, Software and analysis tools, and Visualisation:
Prof Andrew Rambaut 104
Funding acquisition, Metadata curation, Project administration, Samples and logistics, Sequencing and analysis, and Software and analysis tools:
Dr Luke B Snell 12
Leadership and supervision, Metadata curation, Project administration, Samples and logistics, Software and analysis tools, and Visualisation:
Rich Livett 116
Funding acquisition, Leadership and supervision, Metadata curation, Project administration, and Samples and logistics:
Dr Catherine Ludden 20, 70
Funding acquisition, Leadership and supervision, Metadata curation, Samples and logistics, and Sequencing and analysis:
Dr Sally Corden 74 and Dr Eleni Nastouli 96, 95, 30
Funding acquisition, Leadership and supervision, Metadata curation, Sequencing and analysis, and Software and analysis tools:
Dr Gaia Nebbia 12
Funding acquisition, Leadership and supervision, Project administration, Samples and logistics, and Sequencing and analysis:
Ian Johnston 116
Leadership and supervision, Metadata curation, Project administration, Samples and logistics, and Sequencing and analysis:
Prof Katrina Lythgoe 5, Dr M. Estee Torok 19, 20 and Prof Ian G Goodfellow 24
Leadership and supervision, Metadata curation, Project administration, Samples and logistics, and Visualisation:
Dr Jacqui A Prieto 97, 82 and Dr Kordo Saeed 97, 83
Leadership and supervision, Metadata curation, Project administration, Sequencing and analysis, and Software and analysis tools:
Dr David K Jackson 116
Leadership and supervision, Metadata curation, Samples and logistics, Sequencing and analysis, and Visualisation:
Dr Catherine Houlihan 96, 94
Leadership and supervision, Metadata curation, Sequencing and analysis, Software and analysis tools, and Visualisation:
Dr Dan Frampton 94, 95
Metadata curation, Project administration, Samples and logistics, Sequencing and analysis, and Software and analysis tools:
Dr William L Hamilton 19 and Dr Adam A Witney 41
Funding acquisition, Samples and logistics, Sequencing and analysis, and Visualisation:
Dr Giselda Bucca 101
Funding acquisition, Leadership and supervision, Metadata curation, and Project administration:
Dr Cassie F Pope 40, 41
Funding acquisition, Leadership and supervision, Metadata curation, and Samples and logistics:
Dr Catherine Moore 74
Funding acquisition, Leadership and supervision, Metadata curation, and Sequencing and analysis:
Prof Emma C Thomson 53
Funding acquisition, Leadership and supervision, Project administration, and Samples and logistics:
Dr Ewan M Harrison 116, 102
Funding acquisition, Leadership and supervision, Sequencing and analysis, and Visualisation:
Prof Colin P Smith 101
Leadership and supervision, Metadata curation, Project administration, and Sequencing and analysis:
Fiona Rogan 77
Leadership and supervision, Metadata curation, Project administration, and Samples and logistics:
Shaun M Beckwith 6, Abigail Murray 6, Dawn Singleton 6, Dr Kirstine Eastick 37, Dr Liz A Sheridan 98, Paul Randell 99, Dr Leigh M Jackson 105, Dr Cristina V Ariani 116 and Dr Sónia Gonçalves 116
Leadership and supervision, Metadata curation, Samples and logistics, and Sequencing and analysis:
Dr Derek J Fairley 3, 77, Prof Matthew W Loose 18 and Joanne Watkins 74
Leadership and supervision, Metadata curation, Samples and logistics, and Visualisation:
Dr Samuel Moses 25, 106
Leadership and supervision, Metadata curation, Sequencing and analysis, and Software and analysis tools:
Dr Sam Nicholls 43, Dr Matthew Bull 74 and Dr Roberto Amato 116
Leadership and supervision, Project administration, Samples and logistics, and Sequencing and analysis:
Prof Darren L Smith 36, 65, 66
Leadership and supervision, Sequencing and analysis, Software and analysis tools, and Visualisation:
Prof David M Aanensen 14, 116 and Dr Jeffrey C Barrett 116
Metadata curation, Project administration, Samples and logistics, and Sequencing and analysis:
Dr Dinesh Aggarwal 20, 116, 70, Dr James G Shepherd 53, Dr Martin D Curran 71 and Dr Surendra Parmar 71
Metadata curation, Project administration, Sequencing and analysis, and Software and analysis tools:
Dr Matthew D Parker 109
Metadata curation, Samples and logistics, Sequencing and analysis, and Software and analysis tools:
Dr Catryn Williams 74
Metadata curation, Samples and logistics, Sequencing and analysis, and Visualisation:
Dr Sharon Glaysher 68
Metadata curation, Sequencing and analysis, Software and analysis tools, and Visualisation:
Dr Anthony P Underwood 14, 116, Dr Matthew Bashton 36, 65, Dr Nicole Pacchiarini 74, Dr Katie F Loveson 84 and Matthew Byott 95, 96
Project administration, Sequencing and analysis, Software and analysis tools, and Visualisation:
Dr Alessandro M Carabelli 20
Funding acquisition, Leadership and supervision, and Metadata curation:
Dr Kate E Templeton 56, 104
Funding acquisition, Leadership and supervision, and Project administration:
Dr Thushan I de Silva 109, Dr Dennis Wang 109, Dr Cordelia F Langford 116 and John Sillitoe 116
Funding acquisition, Leadership and supervision, and Samples and logistics:
Prof Rory N Gunson 55
Funding acquisition, Leadership and supervision, and Sequencing and analysis:
Dr Simon Cottrell 74, Dr Justin O’Grady 75, 103 and Prof Dominic Kwiatkowski 116, 108
Leadership and supervision, Metadata curation, and Project administration:
Dr Patrick J Lillie 37
Leadership and supervision, Metadata curation, and Samples and logistics:
Dr Nicholas Cortes 33, Dr Nathan Moore 33, Dr Claire Thomas 33, Phillipa J Burns 37, Dr Tabitha W Mahungu 80 and Steven Liggett 86
Leadership and supervision, Metadata curation, and Sequencing and analysis:
Angela H Beckett 13, 81 and Prof Matthew TG Holden 73
Leadership and supervision, Project administration, and Samples and logistics:
Dr Lisa J Levett 34, Dr Husam Osman 70, 35 and Dr Mohammed O Hassan-Ibrahim 99
Leadership and supervision, Project administration, and Sequencing and analysis:
Dr David A Simpson 77
Leadership and supervision, Samples and logistics, and Sequencing and analysis:
Dr Meera Chand 72, Prof Ravi K Gupta 102, Prof Alistair C Darby 107 and Prof Steve Paterson 107
Leadership and supervision, Sequencing and analysis, and Software and analysis tools:
Prof Oliver G Pybus 23, Dr Erik M Volz 39, Prof Daniela de Angelis 52, Prof David L Robertson 53, Dr Andrew J Page 75 and Dr Inigo Martincorena 116
Leadership and supervision, Sequencing and analysis, and Visualisation:
Dr Louise Aigrain 116 and Dr Andrew R Bassett 116
Metadata curation, Project administration, and Samples and logistics:
Dr Nick Wong 50, Dr Yusri Taha 89, Michelle J Erkiert 99 and Dr Michael H Spencer Chapman 116, 102
Metadata curation, Project administration, and Sequencing and analysis:
Dr Rebecca Dewar 56 and Martin P McHugh 56, 111
Metadata curation, Project administration, and Software and analysis tools:
Siddharth Mookerjee 38, 57
Metadata curation, Project administration, and Visualisation:
Stephen Aplin 97, Matthew Harvey 97, Thea Sass 97, Dr Helen Umpleby 97 and Helen Wheeler 97
Metadata curation, Samples and logistics, and Sequencing and analysis:
Dr James P McKenna 3, Dr Ben Warne 9, Joshua F Taylor 22, Yasmin Chaudhry 24, Rhys Izuagbe 24, Dr Aminu S Jahun 24, Dr Gregory R Young 36, 65, Dr Claire McMurray 43, Dr Clare M McCann 65, 66, Dr Andrew Nelson 65, 66 and Scott Elliott 68
Metadata curation, Samples and logistics, and Visualisation:
Hannah Lowe 25
Metadata curation, Sequencing and analysis, and Software and analysis tools:
Dr Anna Price 11, Matthew R Crown 65, Dr Sara Rey 74, Dr Sunando Roy 96 and Dr Ben Temperton 105
Metadata curation, Sequencing and analysis, and Visualisation:
Dr Sharif Shaaban 73 and Dr Andrew R Hesketh 101
Project administration, Samples and logistics, and Sequencing and analysis:
Dr Kenneth G Laing 41, Dr Irene M Monahan 41 and Dr Judith Heaney 95, 96, 34
Project administration, Samples and logistics, and Visualisation:
Dr Emanuela Pelosi 97, Siona Silviera 97 and Dr Eleri Wilson-Davies 97
Samples and logistics, Software and analysis tools, and Visualisation:
Dr Helen Fryer 5
Sequencing and analysis, Software and analysis tools, and Visualization:
Dr Helen Adams 4, Dr Louis du Plessis 23, Dr Rob Johnson 39, Dr William T Harvey 53, 42, Dr Joseph Hughes 53, Dr Richard J Orton 53, Dr Lewis G Spurgin 59, Dr Yann Bourgeois 81, Dr Chris Ruis 102, Áine O'Toole 104, Marina Gourtovaia 116 and Dr Theo Sanderson 116
Funding acquisition, and Leadership and supervision:
Dr Christophe Fraser 5, Dr Jonathan Edgeworth 12, Prof Judith Breuer 96, 29, Dr Stephen L Michell 105 and Prof John A Todd 115
Funding acquisition, and Project administration:
Michaela John 10 and Dr David Buck 115
Leadership and supervision, and Metadata curation:
Dr Kavitha Gajee 37 and Dr Gemma L Kay 75
Leadership and supervision, and Project administration:
Prof Sharon J Peacock 20, 70 and David Heyburn 74
Leadership and supervision, and Samples and logistics:
Katie Kitchman 37, Prof Alan McNally 43, 93, David T Pritchard 50, Dr Samir Dervisevic 58, Dr Peter Muir 70, Dr Esther Robinson 70, 35, Dr Barry B Vipond 70, Newara A Ramadan 78, Dr Christopher Jeanes 90, Danni Weldon 116, Jana Catalan 118 and Neil Jones 118
Leadership and supervision, and Sequencing and analysis:
Dr Ana da Silva Filipe 53, Dr Chris Williams 74, Marc Fuchs 77, Dr Julia Miskelly 77, Dr Aaron R Jeffries 105, Karen Oliver 116 and Dr Naomi R Park 116
Metadata curation, and Samples and logistics:
Amy Ash 1, Cherian Koshy 1, Magdalena Barrow 7, Dr Sarah L Buchan 7, Dr Anna Mantzouratou 7, Dr Gemma Clark 15, Dr Christopher W Holmes 16, Sharon Campbell 17, Thomas Davis 21, Ngee Keong Tan 22, Dr Julianne R Brown 29, Dr Kathryn A Harris 29, 2, Stephen P Kidd 33, Dr Paul R Grant 34, Dr Li Xu-McCrae 35, Dr Alison Cox 38, 63, Pinglawathee Madona 38, 63, Dr Marcus Pond 38, 63, Dr Paul A Randell 38, 63, Karen T Withell 48, Cheryl Williams 51, Dr Clive Graham 60, Rebecca Denton-Smith 62, Emma Swindells 62, Robyn Turnbull 62, Dr Tim J Sloan 67, Dr Andrew Bosworth 70, 35, Stephanie Hutchings 70, Hannah M Pymont 70, Dr Anna Casey 76, Dr Liz Ratcliffe 76, Dr Christopher R Jones 79, 105, Dr Bridget A Knight 79, 105, Dr Tanzina Haque 80, Dr Jennifer Hart 80, Dr Dianne Irish-Tavares 80, Eric Witele 80, Craig Mower 86, Louisa K Watson 86, Jennifer Collins 89, Gary Eltringham 89, Dorian Crudgington 98, Ben Macklin 98, Prof Miren Iturriza-Gomara 107, Dr Anita O Lucaci 107 and Dr Patrick C McClure 113
Metadata curation, and Sequencing and analysis:
Matthew Carlile 18, Dr Nadine Holmes 18, Dr Christopher Moore 18, Dr Nathaniel Storey 29, Dr Stefan Rooke 73, Dr Gonzalo Yebra 73, Dr Noel Craine 74, Malorie Perry 74, Dr Nabil-Fareed Alikhan 75, Dr Stephen Bridgett 77, Kate F Cook 84, Christopher Fearn 84, Dr Salman Goudarzi 84, Prof Ronan A Lyons 88, Dr Thomas Williams 104, Dr Sam T Haldenby 107, Jillian Durham 116 and Dr Steven Leonard 116
Metadata curation, and Software and analysis tools:
Robert M Davies 116
Project administration, and Samples and logistics:
Dr Rahul Batra 12, Beth Blane 20, Dr Moira J Spyer 30, 95, 96, Perminder Smith 32, 112, Mehmet Yavus 85, 109, Dr Rachel J Williams 96, Dr Adhyana IK Mahanama 97, Dr Buddhini Samaraweera 97, Sophia T Girgis 102, Samantha E Hansford 109, Dr Angie Green 115, Dr Charlotte Beaver 116, Katherine L Bellis 116, 102, Matthew J Dorman 116, Sally Kay 116, Liam Prestwood 116 and Dr Shavanthi Rajatileka 116
Project administration, and Sequencing and analysis:
Dr Joshua Quick 43
Project administration, and Software and analysis tools:
Radoslaw Poplawski 43
Samples and logistics, and Sequencing and analysis:
Dr Nicola Reynolds 8, Andrew Mack 11, Dr Arthur Morriss 11, Thomas Whalley 11, Bindi Patel 12, Dr Iliana Georgana 24, Dr Myra Hosmillo 24, Malte L Pinckert 24, Dr Joanne Stockton 43, Dr John H Henderson 65, Amy Hollis 65, Dr William Stanley 65, Dr Wen C Yew 65, Dr Richard Myers 72, Dr Alicia Thornton 72, Alexander Adams 74, Tara Annett 74, Dr Hibo Asad 74, Alec Birchley 74, Jason Coombes 74, Johnathan M Evans 74, Laia Fina 74, Bree Gatica-Wilcox 74, Lauren Gilbert 74, Lee Graham 74, Jessica Hey 74, Ember Hilvers 74, Sophie Jones 74, Hannah Jones 74, Sara Kumziene-Summerhayes 74, Dr Caoimhe McKerr 74, Jessica Powell 74, Georgia Pugh 74, Sarah Taylor 74, Alexander J Trotter 75, Charlotte A Williams 96, Leanne M Kermack 102, Benjamin H Foulkes 109, Marta Gallis 109, Hailey R Hornsby 109, Stavroula F Louka 109, Dr Manoj Pohare 109, Paige Wolverson 109, Peijun Zhang 109, George MacIntyre-Cockett 115, Amy Trebes 115, Dr Robin J Moll 116, Lynne Ferguson 117, Dr Emily J Goldstein 117, Dr Alasdair Maclean 117 and Dr Rachael Tomb 117
Samples and logistics, and Software and analysis tools:
Dr Igor Starinskij 53
Sequencing and analysis, and Software and analysis tools:
Laura Thomson 5, Joel Southgate 11, 74, Dr Moritz UG Kraemer 23, Dr Jayna Raghwani 23, Dr Alex E Zarebski 23, Olivia Boyd 39, Lily Geidelberg 39, Dr Chris J Illingworth 52, Dr Chris Jackson 52, Dr David Pascall 52, Dr Sreenu Vattipally 53, Timothy M Freeman 109, Dr Sharon N Hsu 109, Dr Benjamin B Lindsey 109, Dr Keith James 116, Kevin Lewis 116, Gerry Tonkin-Hill 116 and Dr Jaime M Tovar-Corona 116
Sequencing and analysis, and Visualisation:
MacGregor Cox 20
Software and analysis tools, and Visualisation:
Dr Khalil Abudahab 14, 116, Mirko Menegazzo 14, Ben EW Taylor MEng 14, 116, Dr Corin A Yeats 14, Afrida Mukaddas 53, Derek W Wright 53, Dr Leonardo de Oliveira Martins 75, Dr Rachel Colquhoun 104, Verity Hill 104, Dr Ben Jackson 104, Dr JT McCrone 104, Dr Nathan Medd 104, Dr Emily Scher 104 and Jon-Paul Keatley 116
Leadership and supervision:
Dr Tanya Curran 3, Dr Sian Morgan 10, Prof Patrick Maxwell 20, Prof Ken Smith 20, Dr Sahar Eldirdiri 21, Anita Kenyon 21, Prof Alison H Holmes 38, 57, Dr James R Price 38, 57, Dr Tim Wyatt 69, Dr Alison E Mather 75, Dr Timofey Skvortsov 77 and Prof John A Hartley 96
Metadata curation:
Prof Martyn Guest 11, Dr Christine Kitchen 11, Dr Ian Merrick 11, Robert Munn 11, Dr Beatrice Bertolusso 33, Dr Jessica Lynch 33, Dr Gabrielle Vernet 33, Stuart Kirk 34, Dr Elizabeth Wastnedge 56, Dr Rachael Stanley 58, Giles Idle 64, Dr Declan T Bradley 69, 77, Dr Jennifer Poyner 79 and Matilde Mori 110
Project administration:
Owen Jones 11, Victoria Wright 18, Ellena Brooks 20, Carol M Churcher 20, Mireille Fragakis 20, Dr Katerina Galai 20, 70, Dr Andrew Jermy 20, Sarah Judges 20, Georgina M McManus 20, Kim S Smith 20, Dr Elaine Westwick 20, Dr Stephen W Attwood 23, Dr Frances Bolt 38, 57, Dr Alisha Davies 74, Elen De Lacy 74, Fatima Downing 74, Sue Edwards 74, Lizzie Meadows 75, Sarah Jeremiah 97, Dr Nikki Smith 109 and Luke Foulser 116
Samples and logistics:
Dr Themoula Charalampous 12, 46, Amita Patel 12, Dr Louise Berry 15, Dr Tim Boswell 15, Dr Vicki M Fleming 15, Dr Hannah C Howson-Wells 15, Dr Amelia Joseph 15, Manjinder Khakh 15, Dr Michelle M Lister 15, Paul W Bird 16, Karlie Fallon 16, Thomas Helmer 16, Dr Claire L McMurray 16, Mina Odedra 16, Jessica Shaw 16, Dr Julian W Tang 16, Nicholas J Willford 16, Victoria Blakey 17, Dr Veena Raviprakash 17, Nicola Sheriff 17, Lesley-Anne Williams 17, Theresa Feltwell 20, Dr Luke Bedford 26, Dr James S Cargill 27, Warwick Hughes 27, Dr Jonathan Moore 28, Susanne Stonehouse 28, Laura Atkinson 29, Jack CD Lee 29, Dr Divya Shah 29, Adela Alcolea-Medina 32, 112, Natasha Ohemeng-Kumi 32, 112, John Ramble 32, 112, Jasveen Sehmi 32, 112, Dr Rebecca Williams 33, Wendy Chatterton 34, Monika Pusok 34, William Everson 37, Anibolina Castigador 44, Emily Macnaughton 44, Dr Kate El Bouzidi 45, Dr Temi Lampejo 45, Dr Malur Sudhanva 45, Cassie Breen 47, Dr Graciela Sluga 48, Dr Shazaad SY Ahmad 49, 70, Dr Ryan P George 49, Dr Nicholas W Machin 49, 70, Debbie Binns 50, Victoria James 50, Dr Rachel Blacow 55, Dr Lindsay Coupland 58, Dr Louise Smith 59, Dr Edward Barton 60, Debra Padgett 60, Garren Scott 60, Dr Aidan Cross 61, Dr Mariyam Mirfenderesky 61, Jane Greenaway 62, Kevin Cole 64, Phillip Clarke 67, Nichola Duckworth 67, Sarah Walsh 67, Kelly Bicknell 68, Robert Impey 68, Dr Sarah Wyllie 68, Richard Hopes 70, Dr Chloe Bishop 72, Dr Vicki Chalker 72, Dr Ian Harrison 72, Laura Gifford 74, Dr Zoltan Molnar 77, Dr Cressida Auckland 79, Dr Cariad Evans 85, 109, Dr Kate Johnson 85, 109, Dr David G Partridge 85, 109, Dr Mohammad Raza 85, 109, Paul Baker 86, Prof Stephen Bonner 86, Sarah Essex 86, Leanne J Murray 86, Andrew I Lawton 87, Dr Shirelle Burton-Fanning 89, Dr Brendan AI Payne 89, Dr Sheila Waugh 89, Andrea N Gomes 91, Maimuna Kimuli 91, Darren R Murray 91, Paula Ashfield 92, Dr Donald Dobie 92, Dr Fiona Ashford 93, Dr Angus Best 93, Dr Liam Crawford 93, Dr Nicola Cumley 93, Dr Megan Mayhew 93, Dr Oliver Megram 93, Dr Jeremy Mirza 93, Dr Emma Moles-Garcia 93, Dr Benita Percival 93, Megan Driscoll 96, Leah Ensell 96, Dr Helen L Lowe 96, Laurentiu Maftei 96, Matteo Mondani 96, Nicola J Chaloner 99, Benjamin J Cogger 99, Lisa J Easton 99, Hannah Huckson 99, Jonathan Lewis 99, Sarah Lowdon 99, Cassandra S Malone 99, Florence Munemo 99, Manasa Mutingwende 99, Roberto Nicodemi 99, Olga Podplomyk 99, Thomas Somassa 99, Dr Andrew Beggs 100, Dr Alex Richter 100, Claire Cormie 102, Joana Dias 102, Sally Forrest 102, Dr Ellen E Higginson 102, Mailis Maes 102, Jamie Young 102, Dr Rose K Davidson 103, Kathryn A Jackson 107, Dr Lance Turtle 107, Dr Alexander J Keeley 109, Prof Jonathan Ball 113, Timothy Byaruhanga 113, Dr Joseph G Chappell 113, Jayasree Dey 113, Jack D Hill 113, Emily J Park 113, Arezou Fanaie 114, Rachel A Hilson 114, Geraldine Yaze 114 and Stephanie Lo 116
Sequencing and analysis:
Safiah Afifi 10, Robert Beer 10, Joshua Maksimovic 10, Kathryn McCluggage 10, Karla Spellman 10, Catherine Bresner 11, William Fuller 11, Dr Angela Marchbank 11, Trudy Workman 11, Dr Ekaterina Shelest 13, 81, Dr Johnny Debebe 18, Dr Fei Sang 18, Dr Marina Escalera Zamudio 23, Dr Sarah Francois 23, Bernardo Gutierrez 23, Dr Tetyana I Vasylyeva 23, Dr Flavia Flaviani 31, Dr Manon Ragonnet-Cronin 39, Dr Katherine L Smollett 42, Alice Broos 53, Daniel Mair 53, Jenna Nichols 53, Dr Kyriaki Nomikou 53, Dr Lily Tong 53, Ioulia Tsatsani 53, Prof Sarah O'Brien 54, Prof Steven Rushton 54, Dr Roy Sanderson 54, Dr Jon Perkins 55, Seb Cotton 56, Abbie Gallagher 56, Dr Elias Allara 70, 102, Clare Pearson 70, 102, Dr David Bibby 72, Dr Gavin Dabrera 72, Dr Nicholas Ellaby 72, Dr Eileen Gallagher 72, Dr Jonathan Hubb 72, Dr Angie Lackenby 72, Dr David Lee 72, Nikos Manesis 72, Dr Tamyo Mbisa 72, Dr Steven Platt 72, Katherine A Twohig 72, Dr Mari Morgan 74, Alp Aydin 75, David J Baker 75, Dr Ebenezer Foster-Nyarko 75, Dr Sophie J Prosolek 75, Steven Rudder 75, Chris Baxter 77, Sílvia F Carvalho 77, Dr Deborah Lavin 77, Dr Arun Mariappan 77, Dr Clara Radulescu 77, Dr Aditi Singh 77, Miao Tang 77, Helen Morcrette 79, Nadua Bayzid 96, Marius Cotic 96, Dr Carlos E Balcazar 104, Dr Michael D Gallagher 104, Dr Daniel Maloney 104, Thomas D Stanton 104, Dr Kathleen A Williamson 104, Dr Robin Manley 105, Michelle L Michelsen 105, Dr Christine M Sambles 105, Dr David J Studholme 105, Joanna Warwick-Dugdale 105, Richard Eccles 107, Matthew Gemmell 107, Dr Richard Gregory 107, Dr Margaret Hughes 107, Charlotte Nelson 107, Dr Lucille Rainbow 107, Dr Edith E Vamos 107, Hermione J Webster 107, Dr Mark Whitehead 107, Claudia Wierzbicki 107, Dr Adrienn Angyal 109, Dr Luke R Green 109, Dr Max Whiteley 109, Emma Betteridge 116, Dr Iraad F Bronner 116, Ben W Farr 116, Scott Goodwin 116, Dr Stefanie V Lensing 116, Shane A McCarthy 116, 102, Dr Michael A Quail 116, Diana Rajan 116, Dr Nicholas M Redshaw 116, Carol Scott 116, Lesley Shirley 116 and Scott AJ Thurston 116
Software and analysis tools:
Dr Will Rowe 43, Amy Gaskin 74, Dr Thanh Le-Viet 75, James Bonfield 116, Jennifier Liddle 116 and Andrew Whitwham 116
1 Barking, Havering and Redbridge University Hospitals NHS Trust, 2 Barts Health NHS Trust, 3 Belfast Health & Social Care Trust, 4 Betsi Cadwaladr University Health Board, 5 Big Data Institute, Nuffield Department of Medicine, University of Oxford, 6 Blackpool Teaching Hospitals NHS Foundation Trust, 7 Bournemouth University, 8 Cambridge Stem Cell Institute, University of Cambridge, 9 Cambridge University Hospitals NHS Foundation Trust, 10 Cardiff and Vale University Health Board, 11 Cardiff University, 12 Centre for Clinical Infection and Diagnostics Research, Department of Infectious Diseases, Guy's and St Thomas' NHS Foundation Trust, 13 Centre for Enzyme Innovation, University of Portsmouth, 14 Centre for Genomic Pathogen Surveillance, University of Oxford, 15 Clinical Microbiology Department, Queens Medical Centre, Nottingham University Hospitals NHS Trust, 16 Clinical Microbiology, University Hospitals of Leicester NHS Trust, 17 County Durham and Darlington NHS Foundation Trust, 18 Deep Seq, School of Life Sciences, Queens Medical Centre, University of Nottingham, 19 Department of Infectious Diseases and Microbiology, Cambridge University Hospitals NHS Foundation Trust, 20 Department of Medicine, University of Cambridge, 21 Department of Microbiology, Kettering General Hospital, 22 Department of Microbiology, South West London Pathology, 23 Department of Zoology, University of Oxford, 24 Division of Virology, Department of Pathology, University of Cambridge, 25 East Kent Hospitals University NHS Foundation Trust, 26 East Suffolk and North Essex NHS Foundation Trust, 27 East Sussex Healthcare NHS Trust, 28 Gateshead Health NHS Foundation Trust, 29 Great Ormond Street Hospital for Children NHS Foundation Trust, 30 Great Ormond Street Institute of Child Health (GOS ICH), University College London (UCL), 31 Guy's and St. Thomas’ Biomedical Research Centre, 32 Guy's and St. Thomas’ NHS Foundation Trust, 33 Hampshire Hospitals NHS Foundation Trust, 34 Health Services Laboratories, 35 Heartlands Hospital, Birmingham, 36 Hub for Biotechnology in the Built Environment, Northumbria University, 37 Hull University Teaching Hospitals NHS Trust, 38 Imperial College Healthcare NHS Trust, 39 Imperial College London, 40 Infection Care Group, St George’s University Hospitals NHS Foundation Trust, 41 Institute for Infection and Immunity, St George’s University of London, 42 Institute of Biodiversity, Animal Health & Comparative Medicine, 43 Institute of Microbiology and Infection, University of Birmingham, 44 Isle of Wight NHS Trust, 45 King's College Hospital NHS Foundation Trust, 46 King's College London, 47 Liverpool Clinical Laboratories, 48 Maidstone and Tunbridge Wells NHS Trust, 49 Manchester University NHS Foundation Trust, 50 Microbiology Department, Buckinghamshire Healthcare NHS Trust, 51 Microbiology, Royal Oldham Hospital, 52 MRC Biostatistics Unit, University of Cambridge, 53 MRC-University of Glasgow Centre for Virus Research, 54 Newcastle University, 55 NHS Greater Glasgow and Clyde, 56 NHS Lothian, 57 NIHR Health Protection Research Unit in HCAI and AMR, Imperial College London, 58 Norfolk and Norwich University Hospitals NHS Foundation Trust, 59 Norfolk County Council, 60 North Cumbria Integrated Care NHS Foundation Trust, 61 North Middlesex University Hospital NHS Trust, 62 North Tees and Hartlepool NHS Foundation Trust, 63 North West London Pathology, 64 Northumbria Healthcare NHS Foundation Trust, 65 Northumbria University, 66 NU-OMICS, Northumbria University, 67 Path Links, Northern Lincolnshire and Goole NHS Foundation Trust, 68 Portsmouth Hospitals University NHS Trust, 69 Public Health Agency, Northern Ireland, 70 Public Health England, 71 Public Health England, Cambridge, 72 Public Health England, Colindale, 73 Public Health Scotland, 74 Public Health Wales, 75 Quadram Institute Bioscience, 76 Queen Elizabeth Hospital, Birmingham, 77 Queen's University Belfast, 78 Royal Brompton and Harefield Hospitals, 79 Royal Devon and Exeter NHS Foundation Trust, 80 Royal Free London NHS Foundation Trust, 81 School of Biological Sciences, University of Portsmouth, 82 School of Health Sciences, University of Southampton, 83 School of Medicine, University of Southampton, 84 School of Pharmacy & Biomedical Sciences, University of Portsmouth, 85 Sheffield Teaching Hospitals NHS Foundation Trust, 86 South Tees Hospitals NHS Foundation Trust, 87 Southwest Pathology Services, 88 Swansea University, 89 The Newcastle upon Tyne Hospitals NHS Foundation Trust, 90 The Queen Elizabeth Hospital King's Lynn NHS Foundation Trust, 91 The Royal Marsden NHS Foundation Trust, 92 The Royal Wolverhampton NHS Trust, 93 Turnkey Laboratory, University of Birmingham, 94 University College London Division of Infection and Immunity, 95 University College London Hospital Advanced Pathogen Diagnostics Unit, 96 University College London Hospitals NHS Foundation Trust, 97 University Hospital Southampton NHS Foundation Trust, 98 University Hospitals Dorset NHS Foundation Trust, 99 University Hospitals Sussex NHS Foundation Trust, 100 University of Birmingham, 101 University of Brighton, 102 University of Cambridge, 103 University of East Anglia, 104 University of Edinburgh, 105 University of Exeter, 106 University of Kent, 107 University of Liverpool, 108 University of Oxford, 109 University of Sheffield, 110 University of Southampton, 111 University of St Andrews, 112 Viapath, Guy's and St Thomas' NHS Foundation Trust, and King's College Hospital NHS Foundation Trust, 113 Virology, School of Life Sciences, Queens Medical Centre, University of Nottingham, 114 Watford General Hospital, 115 Wellcome Centre for Human Genetics, Nuffield Department of Medicine, University of Oxford, 116 Wellcome Sanger Institute, 117 West of Scotland Specialist Virology Centre, NHS Greater Glasgow and Clyde, 118 Whittington Health NHS Trust
Contributor Information
Derek W Wright, MRC-University of Glasgow Centre for Virus Research, University of Glasgow, Garscube Campus, 464 Bearsden Road, Glasgow G61 1QH, UK.
Joseph Hughes, MRC-University of Glasgow Centre for Virus Research, University of Glasgow, Garscube Campus, 464 Bearsden Road, Glasgow G61 1QH, UK.
MacGregor Cox, Department of Medicine, University of Cambridge, Addenbrookes Hospital, Hills Road, Cambridge CB2 0QQ, UK.
Thomas P Peacock, Department of Infectious Disease, St Mary’s Medical School, Imperial College London, Praed Street, London, Westminster W2 1NY, UK.
Rachel Colquhoun, Institute of Evolutionary Biology, University of Edinburgh, Charlotte Auerbach Road, Edinburgh EH9 3FL, UK. MRC-University of Glasgow Centre for Virus Research, University of Glasgow, Garscube Campus, 464 Bearsden Road, Glasgow G61 1QH, UK.
Ben Jackson, Institute of Evolutionary Biology, University of Edinburgh, Charlotte Auerbach Road, Edinburgh EH9 3FL, UK.
Richard Orton, MRC-University of Glasgow Centre for Virus Research, University of Glasgow, Garscube Campus, 464 Bearsden Road, Glasgow G61 1QH, UK.
Morten Nielsen, Department of Health Technology, Technical University of Denmark, Lyngby DK-2800, Denmark.
Nienyun Sharon Hsu, The Florey Institute for Host-Pathogen Interactions and Department of Infection, Immunity and Cardiovascular Disease, Medical School, University of Sheffield, Beech Hill Road, Sheffield S10 2RX, UK.
The COVID-19 Genomics UK (COG-UK) consortium, https://www.cogconsortium.uk,Full list of consortium names and affiliations are in.
Ewan M Harrison, Department of Medicine, University of Cambridge, Addenbrookes Hospital, Hills Road, Cambridge CB2 0QQ, UK. Wellcome Sanger Institute, Wellcome Genome Campus, Hinxton CB10 1SA, UK. Department of Public Health and Primary Care, University of Cambridge, Worts Causeway, Cambridge CB1 8RN, UK.
Thushan I de Silva, The Florey Institute for Host-Pathogen Interactions and Department of Infection, Immunity and Cardiovascular Disease, Medical School, University of Sheffield, Beech Hill Road, Sheffield S10 2RX, UK.
Andrew Rambaut, Institute of Evolutionary Biology, University of Edinburgh, Charlotte Auerbach Road, Edinburgh EH9 3FL, UK.
Sharon J Peacock, Department of Medicine, University of Cambridge, Addenbrookes Hospital, Hills Road, Cambridge CB2 0QQ, UK.
David L Robertson, MRC-University of Glasgow Centre for Virus Research, University of Glasgow, Garscube Campus, 464 Bearsden Road, Glasgow G61 1QH, UK.
Alessandro M Carabelli, Department of Medicine, University of Cambridge, Addenbrookes Hospital, Hills Road, Cambridge CB2 0QQ, UK.
Funding
COG-UK is supported by funding from the Medical Research Council (MRC) part of UK Research & Innovation (UKRI), the National Institute of Health Research (NIHR) (MC_PC_19027), and Genome Research Limited, operating as the Wellcome Sanger Institute. W.T.H. and T.P.P. are supported by the G2P-UK National Virology Consortium (MR/W005611/1) and W.T.H. by the MRC (MR/R024758/1). D.L.R., D.W., and J.H. are funded by the MRC (MC_UU_12014/12) and D.L.R. by the Wellcome Trust (220977/Z/20/Z).
References
- Andreano E. et al. (2021) ‘SARS-CoV-2 Escape from a Highly Neutralizing COVID-19 Convalescent Plasma’, Proceedings of the National Academy of Sciences, 118: e2103154118. [Europe PMC free article] [Abstract] [Google Scholar]
- Attali D. (2020), Shinyjs: Easily Improve the User Experience of Your Shiny Apps in Seconds <https://CRAN.R-project.org/package=shinyjs> accessed 16 Mar 2022.
- Barnes C. O. et al. (2020) ‘SARS-CoV-2 Neutralizing Antibody Structures Inform Therapeutic Strategies’, Nature, 588: 682–7. [Europe PMC free article] [Abstract] [Google Scholar]
- Baum A. et al. (2020) ‘Antibody Cocktail to SARS-CoV-2 Spike Protein Prevents Rapid Mutational Escape Seen with Individual Antibodies’, Science, 369: 1014–8. [Europe PMC free article] [Abstract] [Google Scholar]
- Beigel J. H. et al. (2020) ‘Remdesivir for the Treatment of Covid-19 — Final Report’, New England Journal of Medicine, 383: 1813–26. [Europe PMC free article] [Abstract] [Google Scholar]
- Chang W. et al. (2021), Shiny: Web Application Framework for R <https://CRAN.R-project.org/package=shiny> accessed 16 Mar 2022.
- Chang W., and Ribeiro B. B. (2021), Shinydashboard: Create Dashboards with ‘Shiny’ <https://CRAN.R-project.org/package=shinydashboard> accessed 16 Mar 2022.
- CHARMM-GUI Archive . (2021), COVID-19 Proteins Library <https://charmm-gui.org/?doc=archive&lib=covid19%202021> accessed 16 Mar 2022.
- Chi X. et al. (2020) ‘A Neutralizing Human Antibody Binds to the N-terminal Domain of the Spike Protein of SARS-CoV-2’, Science (New York, N.Y.), 369: 650–5. [Europe PMC free article] [Abstract] [Google Scholar]
- Conway J. R. et al. (2017) ‘UpSetR: An R Package for the Visualization of Intersecting Sets and Their Properties’, Bioinformatics, 33: 2938–40. [Europe PMC free article] [Abstract] [Google Scholar]
- Duchene S. et al. (2020) ‘Temporal Signal and the Phylodynamic Threshold of SARS-CoV-2’, Virus Evolution, 6: veaa061. [Europe PMC free article] [Abstract] [Google Scholar]
- Granjon D. (2021). ‘shinydashboardPlus: Add More “AdminLTE2” Components to “shinydashboard”’.
- Greaney A. J. et al. (2021) ‘Comprehensive Mapping of Mutations in the SARS-CoV-2 Receptor-binding Domain that Affect Recognition by Polyclonal Human Plasma Antibodies’, Cell Host & Microbe, 29: 463–76.e466. [Europe PMC free article] [Abstract] [Google Scholar]
- Gu Z. et al. (2016) ‘Complex Heatmaps Reveal Patterns and Correlations in Multidimensional Genomic Data’, Bioinformatics, 32: 2847–9. [Abstract] [Google Scholar]
- Harvey W. T. et al. (2021) ‘SARS-CoV-2 Variants, Spike Mutations and Immune Escape’, Nature Reviews. Microbiology, 19: 409–24. [Europe PMC free article] [Abstract] [Google Scholar]
- Houldcroft C. J. et al. (2017) ‘Clinical and Biological Insights from Viral Genome Sequencing’, Nature Reviews. Microbiology, 15: 183–92. [Europe PMC free article] [Abstract] [Google Scholar]
- Hu B. et al. (2021) ‘Characteristics of SARS-CoV-2 and COVID-19’, Nature Reviews. Microbiology, 19: 141–54. [Europe PMC free article] [Abstract] [Google Scholar]
- Hufsky F. et al. (2021) ‘Computational Strategies to Combat COVID-19: Useful Tools to Accelerate SARS-CoV-2 and Coronavirus Research’, Briefings in Bioinformatics, 22: 642–63. [Europe PMC free article] [Abstract] [Google Scholar]
- Lex A. et al. (2014) ‘UpSet: Visualization of Intersecting Sets’, IEEE Transactions on Visualization and Computer Graphics, 20: 1983–92. [Europe PMC free article] [Abstract] [Google Scholar]
- Li Q. et al. (2020) ‘The Impact of Mutations in SARS-CoV-2 Spike on Viral Infectivity and Antigenicity’, Cell, 182: 1284–94.e1289. [Europe PMC free article] [Abstract] [Google Scholar]
- Liu Z. et al. (2021) ‘Identification of SARS-CoV-2 Spike Mutations that Attenuate Monoclonal and Serum Antibody Neutralization’, Cell Host & Microbe, 29: 477–88.e474. [Europe PMC free article] [Abstract] [Google Scholar]
- MacLean O. A. et al. (2021) ‘Natural Selection in the Evolution of SARS-CoV-2 in Bats Created a Generalist Virus and Highly Capable Human Pathogen’, PLoS Biology, 19: e3001115. [Europe PMC free article] [Abstract] [Google Scholar]
- Martin D. P. et al. (2021) ‘The Emergence and Ongoing Convergent Evolution of the SARS-CoV-2 N501Y Lineages’, Cell, 184: 5189–200.e5187. [Europe PMC free article] [Abstract] [Google Scholar]
- Meredith L. W. et al. (2020) ‘Rapid Implementation of SARS-CoV-2 Sequencing to Investigate Cases of Health-care Associated COVID-19: A Prospective Genomic Surveillance Study’, The Lancet Infectious Diseases, 20: 1263–72. [Europe PMC free article] [Abstract] [Google Scholar]
- Nicholls S. M. et al. (2021) ‘CLIMB-COVID: Continuous Integration Supporting Decentralised Sequencing for SARS-CoV-2 Genomic Surveillance’, Genome Biology, 22: 196. [Europe PMC free article] [Abstract] [Google Scholar]
- O’Toole Á. et al. (2021) ‘Assignment of Epidemiological Lineages in an Emerging Pandemic Using the Pangolin Tool’, Virus Evolution, 7: veab064. [Europe PMC free article] [Abstract] [Google Scholar]
- Perrier V. et al. (2021). ‘shinyWidgets: Custom Inputs Widgets for Shiny’.
- Plotly Technologies Inc . (2015), Collaborative Data Science <https://plot.ly> accessed 16 Mar 2022.
- Rambaut A. et al. (2020) ‘A Dynamic Nomenclature Proposal for SARS-CoV-2 Lineages to Assist Genomic Epidemiology’, Nature Microbiology, 5: 1403–7. [Europe PMC free article] [Abstract] [Google Scholar]
- Reynisson B. et al. (2020) ‘NetMHCpan-4.1 And NetMHCIIpan-4.0: Improved Predictions of MHC Antigen Presentation by Concurrent Motif Deconvolution and Integration of MS MHC Eluted Ligand Data’, Nucleic Acids Research, 48: W449–54. [Europe PMC free article] [Abstract] [Google Scholar]
- Robson F. et al. (2020) ‘Coronavirus RNA Proofreading: Molecular Basis and Therapeutic Targeting’, Molecular Cell, 79: 710–27. [Europe PMC free article] [Abstract] [Google Scholar]
- Schrödinger-LLC (2010) The PyMOL Molecular Graphics System <https://www.pymol.org/> accessed 16 Mar 2022.
- Shu Y., and McCauley J. (2017) ‘GISAID: Global Initiative on Sharing All Influenza Data - from Vision to Reality’, Euro Surveillance : Bulletin European Sur Les Maladies Transmissibles = European Communicable Disease Bulletin, 22: 30494. [Europe PMC free article] [Abstract] [Google Scholar]
- Sidebottom D. B., and Gill D. (2021) ‘Ronapreve for Prophylaxis and Treatment of Covid-19’, BMJ, 374: n2136. [Abstract] [Google Scholar]
- Team, R. C. (2021) R: A Language and Environment for Statistical Computing. R Foundation for Statistical Computing, Vienna, Austria <https://www.R-project.org/> accessed 16 Mar 2022.
- Wagih O. (2017) ‘Ggseqlogo: A Versatile R Package for Drawing Sequence Logos’, Bioinformatics, 33: 3645–7. [Abstract] [Google Scholar]
- Wang Z. et al. (2021) ‘mRNA Vaccine-elicited Antibodies to SARS-CoV-2 and Circulating Variants’, Nature, 592: 616–22. [Europe PMC free article] [Abstract] [Google Scholar]
- Weisblum Y. et al. (2020) ‘Escape from Neutralizing Antibodies by SARS-CoV-2 Spike Protein Variants’, eLife, 9: e61312. [Europe PMC free article] [Abstract] [Google Scholar]
- Wickham H. et al. (2019) ‘Welcome to the Tidyverse’, Journal of Open Source Software, 4: 1686. [Google Scholar]
- Woo H. et al. (2020) ‘Developing a Fully Glycosylated Full-Length SARS-CoV-2 Spike Protein Model in a Viral Membrane’, The Journal of Physical Chemistry. B, 124: 7128–37. [Europe PMC free article] [Abstract] [Google Scholar]
- Wrapp D. et al. (2020) ‘Cryo-EM Structure of the 2019-nCoV Spike in the Prefusion Conformation’, Science, 367: 1260–3. [Europe PMC free article] [Abstract] [Google Scholar]
Articles from Virus Evolution are provided here courtesy of Oxford University Press
Full text links
Read article at publisher's site: https://doi.org/10.1093/ve/veac023
Read article for free, from open access legal sources, via Unpaywall:
https://academic.oup.com/ve/article-pdf/8/1/veac023/43221358/veac023.pdf
Citations & impact
Impact metrics
Citations of article over time
Alternative metrics

Discover the attention surrounding your research
https://www.altmetric.com/details/125235588
Smart citations by scite.ai
Explore citation contexts and check if this article has been
supported or disputed.
https://scite.ai/reports/10.1093/ve/veac023
Article citations
SARS-CoV-2 Genomic Epidemiology Dashboards: A Review of Functionality and Technological Frameworks for the Public Health Response.
Genes (Basel), 15(7):876, 03 Jul 2024
Cited by: 0 articles | PMID: 39062655 | PMCID: PMC11275337
Review Free full text in Europe PMC
Development and application of EpitopeScan, a Python3 toolset for mutation tracking in SARS-CoV-2 immunogenic epitopes.
Front Immunol, 15:1356314, 22 May 2024
Cited by: 0 articles | PMID: 38840924
High-throughput screening of genetic and cellular drivers of syncytium formation induced by the spike protein of SARS-CoV-2.
Nat Biomed Eng, 8(3):291-309, 23 Nov 2023
Cited by: 1 article | PMID: 37996617 | PMCID: PMC10963270
The Effect of Select SARS-CoV-2 N-Linked Glycan and Variant of Concern Spike Protein Mutations on C-Type Lectin-Receptor-Mediated Infection.
Viruses, 15(9):1901, 09 Sep 2023
Cited by: 1 article | PMID: 37766307 | PMCID: PMC10535197
Relative vaccine effectiveness of mRNA COVID-19 boosters in people aged at least 75 years during the spring-summer (monovalent vaccine) and autumn-winter (bivalent vaccine) booster campaigns: a prospective test negative case-control study, United Kingdom, 2022.
Euro Surveill, 28(48), 01 Nov 2023
Cited by: 0 articles | PMID: 38037728 | PMCID: PMC10690860
Go to all (22) article citations
Data
Data behind the article
This data has been text mined from the article, or deposited into data resources.
BioProject
- (1 citation) BioProject - PRJEB37886
Protein structures in PDBe
-
(3 citations)
PDBe - 6VSBView structure
RefSeq - NCBI Reference Sequence Database
- (1 citation) RefSeq - NC_045512
Similar Articles
To arrive at the top five similar articles we use a word-weighted algorithm to compare words from the Title and Abstract of each citation.
The SARS-CoV-2 Spike Protein Mutation Explorer: using an interactive application to improve the public understanding of SARS-CoV-2 variants of concern.
J Vis Commun Med, 46(3):122-132, 01 Jul 2023
Cited by: 0 articles | PMID: 37526402 | PMCID: PMC10726978
The Biological Functions and Clinical Significance of SARS-CoV-2 Variants of Corcern.
Front Med (Lausanne), 9:849217, 20 May 2022
Cited by: 38 articles | PMID: 35669924 | PMCID: PMC9163346
Review Free full text in Europe PMC
Minimal Crossover between Mutations Associated with Omicron Variant of SARS-CoV-2 and CD8+ T-Cell Epitopes Identified in COVID-19 Convalescent Individuals.
mBio, 13(2):e0361721, 01 Mar 2022
Cited by: 64 articles | PMID: 35229637 | PMCID: PMC8941890
Variant-specific deleterious mutations in the SARS-CoV-2 genome reveal immune responses and potentials for prophylactic vaccine development.
Front Pharmacol, 14:1090717, 07 Feb 2023
Cited by: 10 articles | PMID: 36825152 | PMCID: PMC9941545
Evolution of the SARS-CoV-2 omicron variants BA.1 to BA.5: Implications for immune escape and transmission.
Rev Med Virol, 32(5):e2381, 20 Jul 2022
Cited by: 216 articles | PMID: 35856385 | PMCID: PMC9349777
Review Free full text in Europe PMC
Funding
Funders who supported this work.
Academy of Medical Sciences (1)
Grant ID: AMS-CSF4-Torok
G2P-UK National Virology Consortium (1)
Grant ID: MR/W005611/1
Medical Research Council (4)
MRC Centre for Virus Research (CVR): exceptional award
Prof Massimo Palmarini, University of Glasgow
Grant ID: MC_PC_19026
COVID-19 Genomics UK Consortium (COG-UK)
Professor Sharon Peacock, Public Health England
Grant ID: MC_PC_19027
Viral Genomics and Bioinformatics
Professor Andrew Davison, University of Glasgow
Grant ID: MC_UU_12014/12
Using a comparative One Health approach to investigate the structural basis of antigenic variation among human and avian influenza viruses
Dr William Harvey, University of Glasgow
Grant ID: MR/R024758/1
Medical Research Council (MRC) part of UK Research & Innovation (UKRI), the National Institute of Health Research (1)
Grant ID: MC_PC_19027
Wellcome Trust (1)
Grant ID: 220977/Z/20/Z